Nvidia CEO Jensen Huang interview: From the Grace CPU to engineer’s metaverse
feedproxy.google.com – 2021-04-17 18:45:33 – Source link
Join Transform 2021 this July 12-16. Register for the AI event of the year.
Nvidia CEO Jensen Huang delivered a keynote speech this week to 180,000 attendees registered for the GTC 21 online-only conference. And Huang dropped a bunch of news across multiple industries that show just how powerful Nvidia has become.
In his talk, Huang described Nvidia’s work on the Omniverse, a version of the metaverse for engineers. The company is starting out with a focus on the enterprise market, and hundreds of enterprises are already supporting and using it. Nvidia has spent hundreds of millions of dollars on the project, which is based on 3D data-sharing standard Universal Scene Description, originally created by Pixar and later open-sourced. The Omniverse is a place where Nvidia can test self-driving cars that use its AI chips and where all sorts of industries will able to test and design products before they’re built in the physical world.
Nvidia also unveiled its Grace central processing unit (CPU), an AI processor for datacenters based on the Arm architecture. Huang announced new DGX Station mini-sucomputers and said customers will be free to rent them as needed for smaller computing projects. And Nvidia unveiled its BlueField 3 data processing units (DPUs) for datacenter computing alongside new Atlan chips for self-driving cars.
Here’s an edited transcript of Huang’s group interview with the press this week. I asked the first question, and other members of the press asked the rest. Huang talked about everything from what the Omniverse means for the game industry to Nvidia’s plans to acquire Arm for $40 billion.
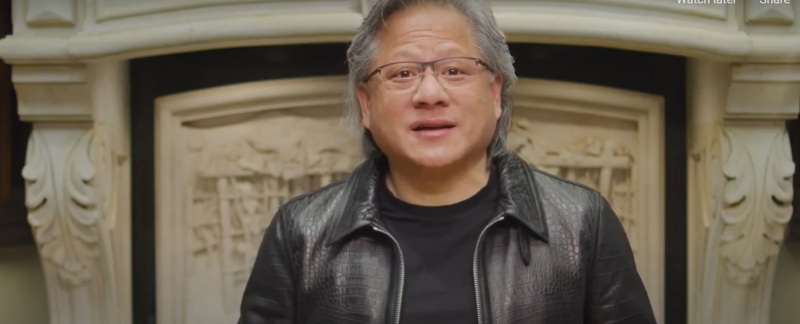
Above: Nvidia CEO Jensen Huang at GTC 21.
Image Credit: Nvidia
Jensen Huang: We had a great GTC. I hope you enjoyed the keynote and some of the talks. We had more than 180,000 registered attendees, 3 times larger than our largest-ever GTC. We had 1,600 talks from some amazing speakers and researchers and scientists. The talks covered a broad range of important topics, from AI [to] 5G, quantum computing, natural language understanding, recommender systems, the most important AI algorithm of our time, self-driving cars, health care, cybersecurity, robotics, edge IOT — the spectrum of topics was stunning. It was very exciting.
Question: I know that the first version of Omniverse is for enterprise, but I’m curious about how you would get game developers to embrace this. Are you hoping or expecting that game developers will build their own versions of a metaverse in Omniverse and eventually try to host consumer metaverses inside Omniverse? Or do you see a different purpose when it’s specifically related to game developers?
Huang: Game development is one of the most complex design pipelines in the world today. I predict that more things will be designed in the virtual world, many of them for games, than there will be designed in the physical world. They will be every bit as high quality and high fidelity, every bit as exquisite, but there will be more buildings, more cars, more boats, more coins, and all of them — there will be so much stuff designed in there. And it’s not designed to be a game prop. It’s designed to be a real product. For a lot of people, they’ll feel that it’s as real to them in the digital world as it is in the physical world.
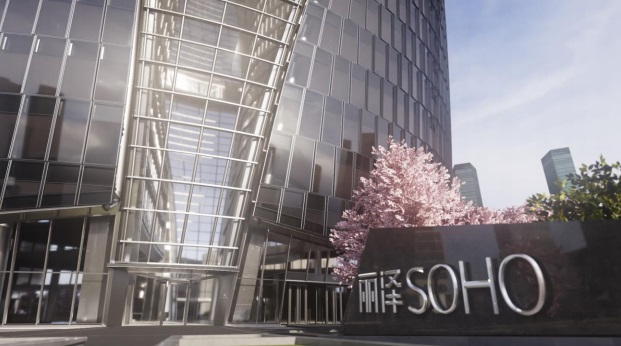
Above: Omniverse lets artists design hotels in a 3D space.
Image Credit: Leeza SOHO, Beijing by ZAHA HADID ARCHITECTS
Omniverse enables game developers working across this complicated pipeline, first of all, to be able to connect. Someone doing rigging for the animation or someone doing textures or someone designing geometry or someone doing lighting, all of these different parts of the design pipeline are complicated. Now they have Omniverse to connect into. Everyone can see what everyone else is doing, rendering in a fidelity that is at the level of what everyone sees. Once the game is developed, they can run it in the Unreal engine that gets exported out. These worlds get run on all kinds of devices. Or Unity. But if someone wants to stream it right out of the cloud, they could do that with Omniverse, because it needs multiple GPUs, a fair amount of computation.
That’s how I see it evolving. But within Omniverse, just the concept of designing virtual worlds for the game developers, it’s going to be a huge benefit to their work flow.
Question: You announced that your current processors target high-performance computing with a special focus on AI. Do you see expanding this offering, developing this CPU line into other segments for computing on a larger scale in the market of datacenters?
Huang: Grace is designed for applications, software that is data-driven. AI is software that writes software. To write that software, you need a lot of experience. It’s just like human intelligence. We need experience. The best way to get that experience is through a lot of data. You can also get it through simulation. For example, the Omniverse simulation system will run on Grace incredibly well. You could simulate — simulation is a form of imagination. You could learn from data. That’s a form of experience. Studying data to infer, to generalize that understanding and turn it into knowledge. That’s what Grace is designed for, these large systems for very important new forms of software, data-driven software.
As a policy, or not a policy, but as a philosophy, we tend not to do anything unless the world needs us to do it and it doesn’t exist. When you look at the Grace architecture, it’s unique. It doesn’t look like anything out there. It solves a problem that didn’t used to exist. It’s an opportunity and a market, a way of doing computing that didn’t exist 20 years ago. It’s sensible to imagine that CPUs that were architected and system architectures that were designed 20 years ago wouldn’t address this new application space. We’ll tend to focus on areas where it didn’t exist before. It’s a new class of problem, and the world needs to do it. We’ll focus on that.
Otherwise, we have excellent partnerships with Intel and AMD. We work very closely with them in the PC industry, in the datacenter, in hyperscale, in supercomputing. We work closely with some exciting new partners. Ampere Computing is doing a great ARM CPU. Marvell is incredible at the edge, 5G systems and I/O systems and storage systems. They’re fantastic there, and we’ll partner with them. We partner with Mediatek, the largest SOC company in the world. These are all companies who have brought great products. Our strategy is to support them. Our philosophy is to support them. By connecting our platform, Nvidia AI or Nvidia RTX, our raytracing platform, with Omniverse and all of our platform technologies to their CPUs, we can expand the overall market. That’s our basic approach. We only focus on building things that the world doesn’t have.
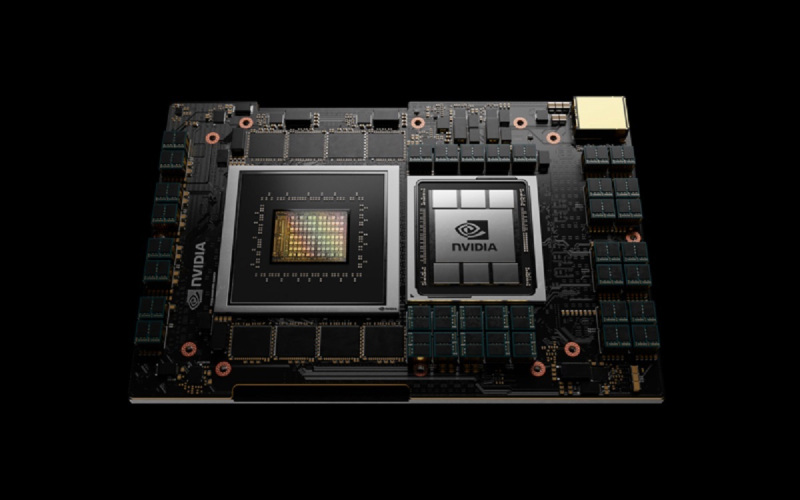
Above: Nvidia’s Grace CPU for datacenters is named after Grace Hopper.
Image Credit: Nvidia
Question: I wanted to follow up on the last question regarding Grace and its use. Does this signal Nvidia’s perhaps ambitions in the CPU space beyond the datacenter? I know you said you’re looking for things that the world doesn’t have yet. Obviously, working with ARM chips in the datacenter space leads to the question of whether we’ll see a commercial version of an Nvidia CPU in the future.
Huang: Our platforms are open. When we build our platforms, we create one version of it. For example, DGX. DGX is fully integrated. It’s bespoke. It has an architecture that’s very specifically Nvidia. It was designed — the first customer was Nvidia researchers. We have a couple billion dollars’ worth of infrastructure our AI researchers are using to develop products and pretrain models and do AI research and self-driving cars. We built DGX primarily to solve a problem we had. Therefore it’s completely bespoke.
We take all of the building blocks, and we open it. We open our computing platform in three layers: the hardware layer, chips and systems; the middleware layer, which is Nvidia AI, Nvidia Omniverse, and it’s open; and the top layer, which is pretrained models, AI skills, like driving skills, speaking skills, recommendation skills, pick and play skills, and so on. We create it vertically, but we architect it and think about it and build it in a way that’s intended for the entire industry to be able to use however they see fit. Grace will be commercial in the same way, just like Nvidia GPUs are commercial.
With respect to its future, our primary preference is that we don’t build something. Our primary preference is that if somebody else is building it, we’re delighted to use it. That allows us to spare our critical resources in the company and focus on advancing the industry in a way that’s rather unique. Advancing the industry in a way that nobody else does. We try to get a sense of where people are going, and if they’re doing a fantastic job at it, we’d rather work with them to bring Nvidia technology to new markets or expand our combined markets together.
The ARM license, as you mentioned — acquiring ARM is a very similar approach to the way we think about all of computing. It’s an open platform. We sell our chips. We license our software. We put everything out there for the ecosystem to be able to build bespoke, their own versions of it, differentiated versions of it. We love the open platform approach.
Question: Can you explain what made Nvidia decide that this datacenter chip was needed right now? Everybody else has datacenter chips out there. You’ve never done this before. How is it different from Intel, AMD, and other datacenter CPUs? Could this cause problems for Nvidia partnerships with those companies, because this puts you in direct competition?
Huang: The answer to the last part — I’ll work my way to the beginning of your question. But I don’t believe so. Companies have leadership that are a lot more mature than maybe given credit for. We compete with the ARM GPUs. On the other hand, we use their CPUs in DGX. Literally, our own product. We buy their CPUs to integrate into our own product — arguably our most important product. We work with the whole semiconductor industry to design their chips into our reference platforms. We work hand in hand with Intel on RTX gaming notebooks. There are almost 80 notebooks we worked on together this season. We advance industry standards together. A lot of collaboration.
Back to why we designed the datacenter CPU, we didn’t think about it that way. The way Nvidia tends to think is we say, “What is a problem that is worthwhile to solve, that nobody in the world is solving and we’re suited to go solve that problem and if we solve that problem it would be a benefit to the industry and the world?” We ask questions literally like that. The philosophy of the company, in leading through that set of questions, finds us solving problems only we will, or only we can, that have never been solved before. The outcome of trying to create a system that can train AI models, language models, that are gigantic, learn from multi-modal data, that would take less than three months — right now, even on a giant supercomputer, it takes months to train 1 trillion parameters. The world would like to train 100 trillion parameters on multi-modal data, looking at video and text at the same time.
The journey there is not going to happen by using today’s architecture and making it bigger. It’s just too inefficient. We created something that is designed from the ground up to solve this class of interesting problems. Now this class of interesting problems didn’t exist 20 years ago, as I mentioned, or even 10 or five years ago. And yet this class of problems is important to the future. AI that’s conversational, that understands language, that can be adapted and pretrained to different domains, what could be more important? It could be the ultimate AI. We came to the conclusion that hundreds of companies are going to need giant systems to pretrain these models and adapt them. It could be thousands of companies. But it wasn’t solvable before. When you have to do computing for three years to find a solution, you’ll never have that solution. If you can do that in weeks, that changes everything.
That’s how we think about these things. Grace is designed for giant-scale data-driven software development, whether it’s for science or AI or just data processing.
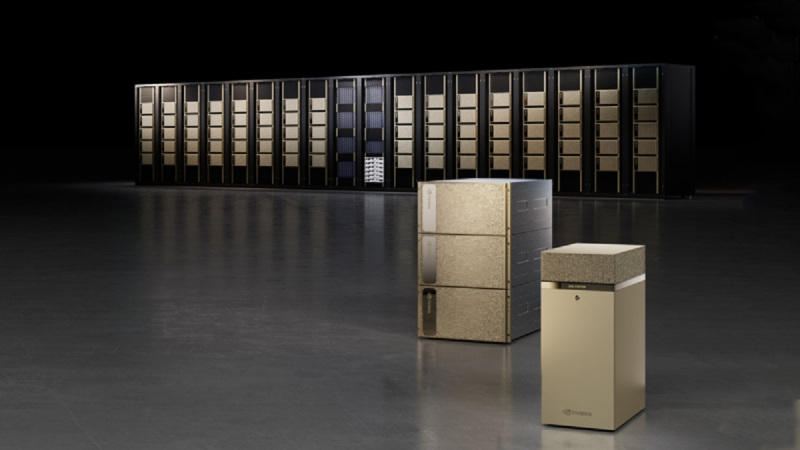
Above: Nvidia DGX SuperPod
Image Credit: Nvidia
Question: You’re proposing a software library for quantum computing. Are you working on hardware components as well?
Huang: We’re not building a quantum computer. We’re building an SDK for quantum circuit simulation. We’re doing that because in order to invent, to research the future of computing, you need the fastest computer in the world to do that. Quantum computers, as you know, are able to simulate exponential complexity problems, which means that you’re going to need a really large computer very quickly. The size of the simulations you’re able to do to verify the results of the research you’re doing to do development of algorithms so you can run them on a quantum computer someday, to discover algorithms — at the moment, there aren’t that many algorithms you can run on a quantum computer that prove to be useful. Grover’s is one of them. Shore’s is another. There are some examples in quantum chemistry.
We give the industry a platform by which to do quantum computing research in systems, in circuits, in algorithms, and in the meantime, in the next 15-20 years, while all of this research is happening, we have the benefit of taking the same SDKs, the same computers, to help quantum chemists do simulations much more quickly. We could put the algorithms to use even today.
And then last, quantum computers, as you know, have incredible exponential complexity computational capability. However, it has extreme I/O limitations. You communicate with it through microwaves, through lasers. The amount of data you can move in and out of that computer is very limited. There needs to be a classical computer that sits next to a quantum computer, the quantum accelerator if you can call it that, that pre-processes the data and does the post-processing of the data in chunks, in such a way that the classical computer sitting next to the quantum computer is going to be super fast. The answer is fairly sensible, that the classical computer will likely be a GPU-accelerated computer.
There are lots of reasons we’re doing this. There are 60 research institutes around the world. We can work with every one of them through our approach. We intend to. We can help every one of them advance their research.
Question: So many workers have moved to work from home, and we’ve seen a huge increase in cybercrime. Has that changed the way AI is used by companies like yours to provide defenses? Are you worried about these technologies in the hands of bad actors who can commit more sophisticated and damaging crimes? Also, I’d love to hear your thoughts broadly on what it will take to solve the chip shortage problem on a lasting global basis.
Huang: The best way is to democratize the technology, in order to enable all of society, which is vastly good, and to put great technology in their hands so that they can use the same technology, and ideally superior technology, to stay safe. You’re right that security is a real concern today. The reason for that is because of virtualization and cloud computing. Security has become a real challenge for companies because every computer inside your datacenter is now exposed to the outside. In the past, the doors to the datacenter were exposed, but once you came into the company, you were an employee, or you could only get in through VPN. Now, with cloud computing, everything is exposed.
The other reason why the datacenter is exposed is because the applications are now aggregated. It used to be that the applications would run monolithically in a container, in one computer. Now the applications for scaled out architectures, for good reasons, have been turned into micro-services that scale out across the whole datacenter. The micro-services are communicating with each other through network protocols. Wherever there’s network traffic, there’s an opportunity to intercept. Now the datacenter has billions of ports, billions of virtual active ports. They’re all attack surfaces.
The answer is you have to do security at the node. You have to start it at the node. That’s one of the reasons why our work with BlueField is so exciting to us. Because it’s a network chip, it’s already in the computer node, and because we invented a way to put high-speed AI processing in an enterprise datacenter — it’s called EGX — with BlueField on one end and EGX on the other, that’s a framework for security companies to build AI. Whether it’s a Check Point or a Fortinet or Palo Alto Networks, and the list goes on, they can now develop software that runs on the chips we build, the computers we build. As a result, every single packet in the datacenter can be monitored. You would inspect every packet, break it down, turn it into tokens or words, read it using natural language understanding, which we talked about a second ago — the natural language understanding would determine whether there’s a particular action that’s needed, a security action needed, and send the security action request back to BlueField.
This is all happening in real time, continuously, and there’s just no way to do this in the cloud because you would have to move way too much data to the cloud. There’s no way to do this on the CPU because it takes too much energy, too much compute load. People don’t do it. I don’t think people are confused about what needs to be done. They just don’t do it because it’s not practical. But now, with BlueField and EGX, it’s practical and doable. The technology exists.
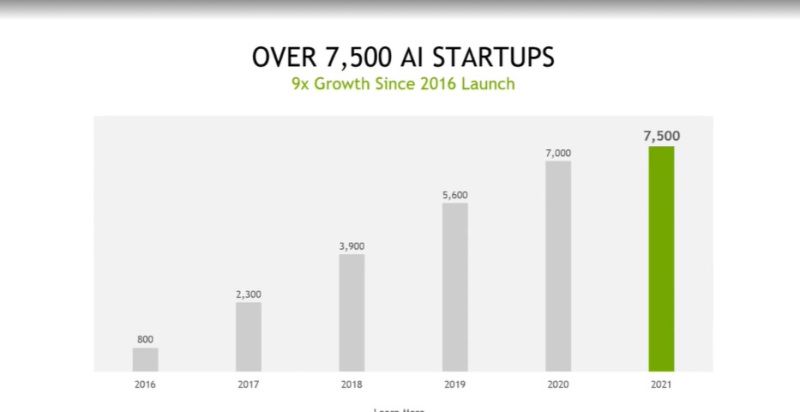
Above: Nvidia’s Inception AI statups over the years.
Image Credit: Nvidia
The second question has to do with chip supply. The industry is caught by a couple of dynamics. Of course one of the dynamics is COVID exposing, if you will, a weakness in the supply chain of the automotive industry, which has two main components it builds into cars. Those main components go through various supply chains, so their supply chain is super complicated. When it shut down abruptly because of COVID, the recovery process was far more complicated, the restart process, than anybody expected. You could imagine it, because the supply chain is so complicated. It’s very clear that cars could be rearchitected, and instead of thousands of components, it wants to be a few centralized components. You can keep your eyes on four things a lot better than a thousand things in different places. That’s one factor.
The other factor is a technology dynamic. It’s been expressed in a lot of different ways, but the technology dynamic is basically that we’re aggregating computing into the cloud, and into datacenters. What used to be a whole bunch of electronic devices — we can now virtualize it, put it in the cloud, and remotely do computing. All the dynamics we were just talking about that have created a security challenge for datacenters, that’s also the reason why these chips are so large. When you can put computing in the datacenter, the chips can be as large as you want. The datacenter is big, a lot bigger than your pocket. Because it can be aggregated and shared with so many people, it’s driving the adoption, driving the pendulum toward very large chips that are very advanced, versus a lot of small chips that are less advanced. All of a sudden, the world’s balance of semiconductor consumption tipped toward the most advanced of computing.
The industry now recognizes this, and surely the world’s largest semiconductor companies recognize this. They’ll build out the necessary capacity. I doubt it will be a real issue in two years because smart people now understand what the problems are and how to address them.
Question: I’d like to know more about what clients and industries Nvidia expects to reach with Grace, and what you think is the size of the market for high-performance datacenter CPUs for AI and advanced computing.
Huang: I’m going to start with I don’t know. But I can give you my intuition. 30 years ago, my investors asked me how big the 3D graphics was going to be. I told them I didn’t know. However, my intuition was that the killer app would be video games, and the PC would become — at the time the PC didn’t even have sound. You didn’t have LCDs. There was no CD-ROM. There was no internet. I said, “The PC is going to become a consumer product. It’s very likely that the new application that will be made possible, that wasn’t possible before, is going to be a consumer product like video games.” They said, “How big is that market going to be?” I said, “I think every human is going to be a gamer.” I said that about 30 years ago. I’m working toward being right. It’s surely happening.
Ten years ago someone asked me, “Why are you doing all this stuff in deep learning? Who cares about detecting cats?” But it’s not about detecting cats. At the time I was trying to detect red Ferraris, as well. It did it fairly well. But anyway, it wasn’t about detecting things. This was a fundamentally new way of developing software. By developing software this way, using networks that are deep, which allows you to capture very high dimensionality, it’s the universal function approximator. If you gave me that, I could use it to predict Newton’s law. I could use it to predict anything you wanted to predict, given enough data. We invested tens of billions behind that intuition, and I think that intuition has proven right.
I believe that there’s a new scale of computer that needs to be built, that needs to learn from basically Earth-scale amounts of data. You’ll have sensors that will be connected to everywhere on the planet, and we’ll use them to predict climate, to create a digital twin of Earth. It’ll be able to predict weather everywhere, anywhere, down to a square meter, because it’s learned the physics and all the geometry of the Earth. It’s learned all of these algorithms. We could do that for natural language understanding, which is extremely complex and changing all the time. The thing people don’t realize about language is it’s evolving continuously. Therefore, whatever AI model you use to understand language is obsolete tomorrow, because of decay, what people call model drift. You’re continuously learning and drifting, if you will, with society.
There’s some very large data-driven science that needs to be done. How many people need language models? Language is thought. Thought is humanity’s ultimate technology. There are so many different versions of it, different cultures and languages and technology domains. How people talk in retail, in fashion, in insurance, in financial services, in law, in the chip industry, in the software industry. They’re all different. We have to train and adapt models for every one of those. How many versions of those? Let’s see. Take 70 languages, multiply by 100 industries that need to use giant systems to train on data forever. That’s maybe an intuition, just to give a sense of my intuition about it. My sense is that it will be a very large new market, just as GPUs were once a zero billion dollar market. That’s Nvidia’s style. We tend to go after zero billion dollar markets, because that’s how we make a contribution to the industry. That’s how we invent the future.
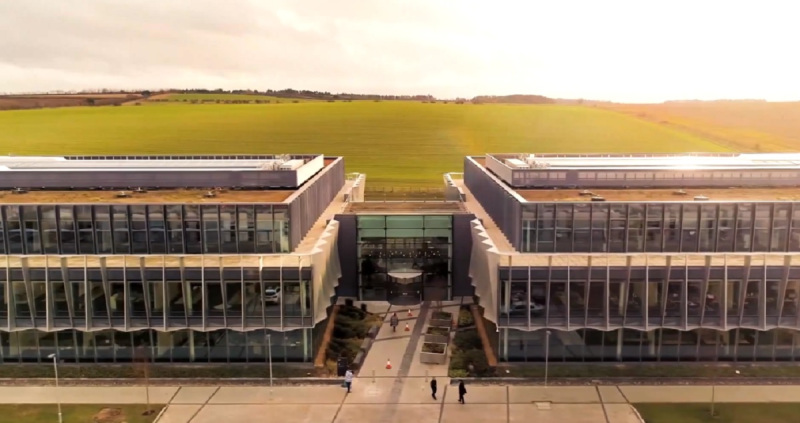
Above: Arm’s campus in Cambridge, United Kingdom.
Image Credit: Arm
Question: Are you still confident that the ARM deal will gain approval by close? With the announcement of Grace and all the other ARM-relevant partnerships you have in development, how important is the ARM acquisition to the company’s goals, and what do you get from owning ARM that you don’t get from licensing?
Huang: ARM and Nvidia are independently and separately excellent businesses, as you know well. We will continue to have excellent separate businesses as we go through this process. However, together we can do many things, and I’ll come back to that. To the beginning of your question, I’m very confident that the regulators will see the wisdom of the transaction. It will provide a surge of innovation. It will create new options for the marketplace. It will allow ARM to be expanded into markets that otherwise are difficult for them to reach themselves. Like many of the partnerships I announced, those are all things bringing AI to the ARM ecosystem, bringing Nvidia’s accelerated computing platform to the ARM ecosystem — it’s something only we and a bunch of computing companies working together can do. The regulators will see the wisdom of it, and our discussions with them are as expected and constructive. I’m confident that we’ll still get the deal done in 2022, which is when we expected it in the first place, about 18 months.
With respect to what we can do together, I demonstrated one example, an early example, at GTC. We announced partnerships with Amazon to combine the Graviton architecture with Nvidia’s GPU architecture to bring modern AI and modern cloud computing to the cloud for ARM. We did that for Ampere computing, for scientific computing, AI in scientific computing. We announced it for Marvell, for edge and cloud platforms and 5G platforms. And then we announced it for Mediatek. These are things that will take a long time to do, and as one company we’ll be able to do it a lot better. The combination will enhance both of our businesses. On the one hand, it expands ARM into new computing platforms that otherwise would be difficult. On the other hand, it expands Nvidia’s AI platform into the ARM ecosystem, which is underexposed to Nvidia’s AI and accelerated computing platform.
Question: I covered Atlan a little more than the other pieces you announced. We don’t really know the node side, but the node side below 10nm is being made in Asia. Will it be something that other countries adopt around the world, in the West? It raises a question for me about the long-term chip supply and the trade issues between China and the United States. Because Atlan seems to be so important to Nvidia, how do you project that down the road, in 2025 and beyond? Are things going to be handled, or not?
Huang: I have every confidence that it will not be an issue. The reason for that is because Nvidia qualifies and works with all of the major foundries. Whatever is necessary to do, we’ll do it when the time comes. A company of our scale and our resources, we can surely adapt our supply chain to make our technology available to customers that use it.
Question: In reference to BlueField 3, and BlueField 2 for that matter, you presented a strong proposition in terms of offloading workloads, but could you provide some context into what markets you expect this to take off in, both right now and going into the future? On top of that, what barriers to adoption remain in the market?
Huang: I’m going to go out on a limb and make a prediction and work backward. Number one, every single datacenter in the world will have an infrastructure computing platform that is isolated from the application platform in five years. Whether it’s five or 10, hard to say, but anyway, it’s going to be complete, and for very logical reasons. The application that’s where the intruder is, you don’t want the intruder to be in a control mode. You want the two to be isolated. By doing this, by creating something like BlueField, we have the ability to isolate.
Second, the processing necessary for the infrastructure stack that is software-defined — the networking, as I mentioned, the east-west traffic in the datacenter, is off the charts. You’re going to have to inspect every single packet now. The east-west traffic in the data center, the packet inspection, is going to be off the charts. You can’t put that on the CPU because it’s been isolated onto a BlueField. You want to do that on BlueField. The amount of computation you’ll have to accelerate onto an infrastructure computing platform is quite significant, and it’s going to get done. It’s going to get done because it’s the best way to achieve zero trust. It’s the best way that we know of, that the industry knows of, to move to the future where the attack surface is basically zero, and yet every datacenter is virtualized in the cloud. That journey requires a reinvention of the datacenter, and that’s what BlueField does. Every datacenter will be outfitted with something like BlueField.
I believe that every single edge device will be a datacenter. For example, the 5G edge will be a datacenter. Every cell tower will be a datacenter. It’ll run applications, AI applications. These AI applications could be hosting a service for a client or they could be doing AI processing to optimize radio beams and strength as the geometry in the environment changes. When traffic changes and the beam changes, the beam focus changes, all of that optimization, incredibly complex algorithms, wants to be done with AI. Every base station is going to be a cloud native, orchestrated, self-optimizing sensor. Software developers will be programming it all the time.
Every single car will be a datacenter. Every car, truck, shuttle will be a datacenter. Every one of those datacenters, the application plane, which is the self-driving car plane, and the control plane, that will be isolated. It’ll be secure. It’ll be functionally safe. You need something like BlueField. I believe that every single edge instance of computing, whether it’s in a warehouse, a factory — how could you have a several-billion-dollar factory with robots moving around and that factory is literally sitting there and not have it be completely tamper-proof? Out of the question, absolutely. That factory will be built like a secure datacenter. Again, BlueField will be there.
Everywhere on the edge, including autonomous machines and robotics, every datacenter, enterprise or cloud, the control plane and the application plane will be isolated. I promise you that. Now the question is, “How do you go about doing it? What’s the obstacle?” Software. We have to port the software. There’s two pieces of software, really, that need to get done. It’s a heavy lift, but we’ve been lifting it for years. One piece is for 80% of the world’s enterprise. They all run VMware vSphere software-defined datacenter. You saw our partnership with VMware, where we’re going to take vSphere stack — we have this, and it’s in the process of going into production now, going to market now … taking vSphere and offloading it, accelerating it, isolating it from the application plane.
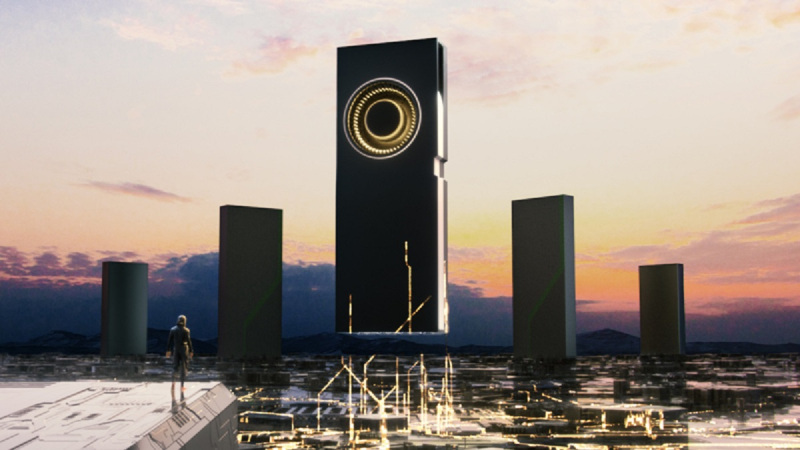
Above: Nvidia has eight new RTX GPU cards.
Image Credit: Nvidia
Number two, for everybody else out at the edge, the telco edge, with Red Hat, we announced a partnership with them, and they’re doing the same thing. Third, for all the cloud service providers who have bespoke software, we created an SDK called DOCA 1.0. It’s released to production, announced at GTC. With this SDK, everyone can program the BlueField, and by using DOCA 1.0, everything they do on BlueField runs on BlueField 3 and BlueField 4. I announced the architecture for all three of those will be compatible with DOCA. Now the software developers know the work they do will be leveraged across a very large footprint, and it will be protected for decades to come.
We had a great GTC. At the highest level, the way to think about that is the work we’re doing is all focused on driving some of the fundamental dynamics happening in the industry. Your questions centered around that, and that’s fantastic. There are five dynamics highlighted during GTC. One of them is accelerated computing as a path forward. It’s the approach we pioneered three decades ago, the approach we strongly believe in. It’s able to solve some challenges for computing that are now front of mind for everyone. The limits of CPUs and their ability to scale to reach some of the problems we’d like to address are facing us. Accelerated computing is the path forward.
Second, to be mindful about the power of AI that we all are excited about. We have to realize that it’s a software that is writing software. The computing method is different. On the other hand, it creates incredible new opportunities. Thinking about the datacenter not just as a big room with computers and network and security appliances, but thinking of the entire datacenter as one computing unit. The datacenter is the new computing unit.
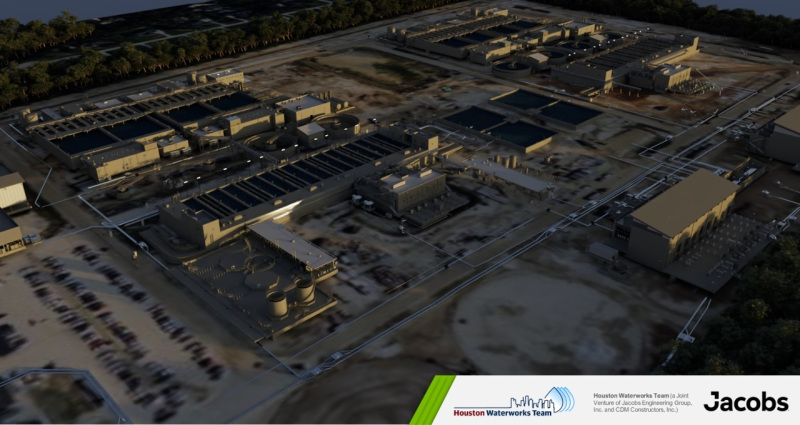
Above: Bentley’s tools used to create a digital twin of a location in the Omniverse.
Image Credit: Nvidia
5G is super exciting to me. Commercial 5G, consumer 5G is exciting. However, it’s incredibly exciting to look at private 5G, for all the applications we just looked at. AI on 5G is going to bring the smartphone moment to agriculture, to logistics, to manufacturing. You can see how excited BMW is about the technologies we’ve put together that allow them to revolutionize the way they do manufacturing, to become much more of a technology company going forward.
Last, the era of robotics is here. We’re going to see some very rapid advances in robotics. One of the critical needs of developing robotics and training robotics, because they can’t be trained in the physical world while they’re still clumsy — we need to give it a virtual world where it can learn how to be a robot. These virtual worlds will be so realistic that they’ll become the digital twins of where the robot goes into production. We spoke about the digital twin vision. PTC is a great example of a company that also sees the vision of this. This is going to be a realization of a vision that’s been talked about for some time. The digital twin idea will be made possible because of technologies that have emerged out of gaming. Gaming and scientific computing have fused together into what we call Omniverse.
GamesBeat
GamesBeat’s creed when covering the game industry is “where passion meets business.” What does this mean? We want to tell you how the news matters to you — not just as a decision-maker at a game studio, but also as a fan of games. Whether you read our articles, listen to our podcasts, or watch our videos, GamesBeat will help you learn about the industry and enjoy engaging with it.
How will you do that? Membership includes access to:
- Newsletters, such as DeanBeat
- The wonderful, educational, and fun speakers at our events
- Networking opportunities
- Special members-only interviews, chats, and “open office” events with GamesBeat staff
- Chatting with community members, GamesBeat staff, and other guests in our Discord
- And maybe even a fun prize or two
- Introductions to like-minded parties
Become a member